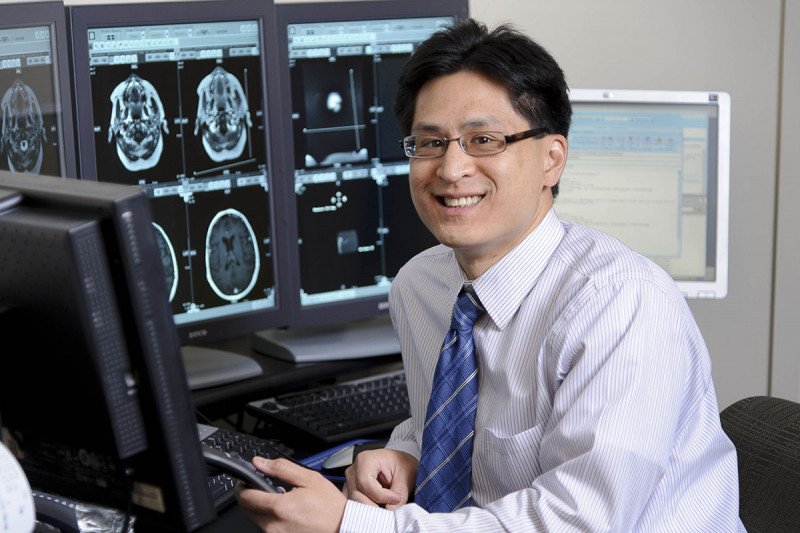
Incorporating radiomics with methyl guanine methyl transferase (MGMT) status can more accurately stratify glioblastoma patients into groups with different survival risks compared to using either prediction tool in isolation, according to the results of our retrospective research published recently in the journal Oncotarget.
Moreover, our methods could easily be used in clinical practice across institutions.
Glioblastoma multiforme (GBM) is the most common malignant central nervous system tumor, and MGMT promoter hypermethylation in this tumor has been shown to be associated with better prognosis. We evaluated the capacity of radiomics features to add complementary information to MGMT status and improve the ability to predict prognosis.
Our study differed from typical radiogenomic studies, which aim to find correlations between radiomics features with genotypic expressions. Instead, we examined the synergistic value of adding radiomics to MGMT methylation status to predict prognosis.
Our results highlight that radiomics features possibly contain information that is not supplied by molecular markers, such as MGMT methylation alone. Particularly, we identified that the methylated MGMT patients can be split into two groups with significantly different survival times.
We built a machine-learning model that identified eight relevant radiomic features from a total of 286 features and then determined their association with overall survival using MRI images of 159 patients with glioblastoma before they received treatment at Memorial Sloan Kettering Cancer Center. (1)
The analysis also identified the group of patients with the best prognosis — a survival probability of 0.61 after 43 months (p=0.0005). These patients had a positive MGMT status and a high negative skewness of Gabor edges. (1)
Gabor wavelet analysis is used to extract the texture features of magnetic resonance tumor images to differentiate between primary central nervous system lymphoma and GBM. Gabor edges are directional sensitive edge detectors, and large negative skewness indicates that GBM patients with longer overall survival (OS) will exhibit a more homogeneous distribution of edges in their tumors compared with those with shorter OS.
In the present study, patients who had both MGMT methylation and large negative skewness of Gabor edge enhancement had a significantly longer median OS of 22.7 months compared to 12.2 months for patients who did not have those characteristics (p=0.004). (1)
Our goal was to build an easy-to-compute and interpretable model that could be used in a clinical practice across institutions based on MGMT status, which is widely available via polymerase chain reaction lab test methods. While next-generation sequencing that reveals underlying tumor genotypic and transcriptomic profiles is showing great promise for predictive utility in many cancers, it is not available at all centers.
Glioblastoma and MGMT Methylation Status
Glioblastoma accounts for about 47 percent of brain cancer cases. It is a primary brain cancer that arises in astrocytes and can spread quickly throughout the brain. GBM is considered a grade IV tumor; however, it is not categorized by stage. It has a poor prognosis of only 5.5 percent survival at five years. (2)Standard therapy includes surgical resection followed by radiotherapy with concomitant and adjuvant chemotherapy with temozolomide. (2)
Recently, scientists have identified several molecular biomarkers in brain cancers that can help predict prognosis and outcomes, and guide decisions for personalized therapies. (3), (4)For glioblastoma, hypermethylation of the MGMT promoter (O6-methylguanine-DNA methyltransferase), which silences the DNA repair enzyme, is associated with better prognosis and better response to temozolomide. (5), (6)
Radiomics
Radiomics involves extracting quantitative feature descriptors of tumor appearance from edges, textures, shape, and histogram features in medical images and performing high-throughput computer analysis. Radiomics has been used to successfully predict outcomes in several cancers (7), (8), (9) and has shown feasibility to predict outcomes, including survival in brain cancer. (1)
Our analysis consisted of several steps. For the training cohort, we included 286 MRI-radiomics features in the model, and it selected the following eight features as relevant to outcome: (1)
- one feature from fluid attenuation inversion recovery images (intensity histogram feature category: interquartile range)
- two features from T1-weighted (T1W1) images (Gray-Level Co-occurrence Matrix feature category: cluster performance and difference variance)
- two features from the contrast-enhanced T1-weighted images (T1W1CE) (Gabor feature category: Gq=0, f=2 skewness and Gq=0, f=2 mean)
- one feature from the T1W1CE images (intensity histogram feature category: 10th percentile)
- two shape features (sphericity and surface-to-volume ratio)
After evaluating the prognostic value of combining MGMT status and radiomics features for predicting overall survival with a training data set (n=98), we validated the model with an independent validation data set (n=61). (1)
Study Results
In the training cohort, MGMT methylation status and Gq=0, f=2 skewness were found to be significant prognostic factors. The combination of these two biomarkers allows the identification of a group of patients with a survival probability of 0.61 after 43 months of observation versus 0.15 for the other patients. (1)
Using the same optimal thresholds identified in the training data set, the Gq=0, f=2 skewness feature from T1W1CE images stayed significantly associated with overall survival. Similar to the training data set, patients in the validation data set who had the highest edge enhancement on T1W1CE (Gq=0, f=2 Skewness < optimal threshold (-0.49; p=0.04) had a longer median survival of 20.6 months compared to 12.2 months for the group with low edge enhancement. (1)
The validation data set confirmed the results of the training data set: Patients who had both MGMT methylation and high negative skewness of Gabor edge enhancement had a significantly longer median overall survival of 22.7 months compared to 12.2 months for patients who did not have those characteristics (p=0.004). (1)
Advancing Predictions and Outcomes in Glioblastoma
Overall, our results suggest that radiomic features likely contain information that cannot be captured by molecular markers alone. In future studies, we plan to focus on methylated MGMT glioblastoma patients and find ways for radiologists to determine visual analogs for the radiomic features that have the most predictive value.
At MSK, the scientists and clinicians in our Brain Tumor Center are dedicated to deploying all available modern genomic and transcriptomic technologies and developing tools to advance new treatment approaches for patients with brain tumors.
For example, we have established a comprehensive patient-derived xenograft program to curate glioblastoma samples that will be used to develop xenografts for comprehensive molecular profiling. We are also establishing a biobank of primary cultures that we plan to mine for genomic insights.
We are currently conducting 24 clinical trials, testing novel approaches in chemotherapy, radiation, and immunotherapy to find new ways to improve outcomes for patients with primary brain cancers.