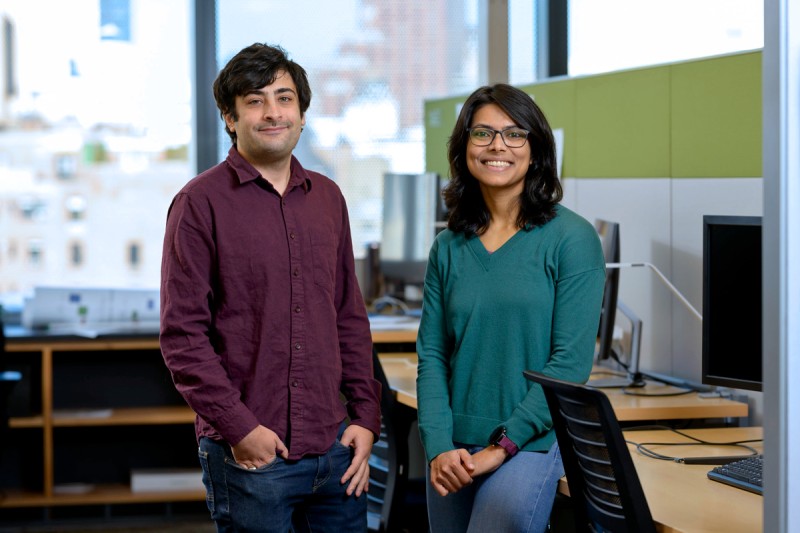
Marie-Josée Kravis Quantitative Biology Fellows Corey Weistuch and Sneha Mitra
These days Sneha Mitra, PhD, spends a lot of time thinking about T cells — a type of white blood cell that helps protect the body from infection and cancer.
A postdoctoral fellow at the Sloan Kettering Institute, Dr. Mitra is working to understand how the interactions and activities of T cells might prevent immunotherapy treatments from benefiting more patients with colorectal cancer.
While immunotherapy can be a godsend for some, the revolutionary approach, which uses a person’s own immune system to fight cancer, doesn’t yet work for everyone. And some types of cancer are particularly resistant.
“I study a type of colorectal cancer called DNA mismatch repair-proficient, or pMMR, which is the normal state our cells are in where the mismatch repair pathway is working properly,” she says. “Immune checkpoint inhibitors don’t work well against this type of cancer. Understanding why is the next step toward improving treatments.”
Rather than seeking answers in beakers and petri dishes, Dr. Mitra’s tools of choice are the keyboard and mouse. By training, Dr. Mitra is a computer scientist. She’s part of a vanguard of researchers known as quantitative biologists who are working to bridge the gap between the fluid, organic world of biology and the orderly, math-heavy world of computer science, physics, and engineering. Their arsenal: data models, statistical analyses, and machine learning — a type of artificial intelligence whose abilities become more refined with training.
A Growing Need for Computational Biologists Who Specialize in Cancer
Dr. Mitra is a recipient of a prestigious Marie-Josée Kravis Fellowship in Quantitative Biology. The fellowship was founded in 2021 through the support of Marie-Josée and Henry R. Kravis. Their generosity and leadership are exemplified by Marie-Josée Kravis’ service as Vice Chair of the Board of Memorial Sloan Kettering Cancer Center (MSK) and Chair of the Board at the Sloan Kettering Institute. Over the years, the Kravises have directed their support toward the future of cancer research as well as to the immediate, practical concerns of researchers.
The Kravis Fellowship in Quantitative Biology was created to address the growing need for computational biologists who specialize in cancer and to foster high-risk, high-impact projects that might otherwise go unfunded.
“We want to train not just a new generation of quantitative biologists working on the most important questions in cancer, but also to develop the next generation of leaders in the field,” says Joan Massagué, PhD, Director of the Sloan Kettering Institute, which serves as a hub for basic and translational research within MSK.
The two-year fellowship, which is accepting new applications through June 21, 2023, has one innovative feature that sets it apart from most other programs: a joint mentorship model. Fellows work with both a “dry lab” devoted to computational science and a “wet lab” focused on cancer biology.
“This unique approach complements MSK’s longtime strengths in discovery science and translational research, and opens enormous potential for these fellows to drive innovations with real clinical impact,” Dr. Massagué says.
Demand for quantitative biologists is high — and the need for quantitative biologists who specialize in cancer is even greater, adds Ushma Neill, PhD, Vice President of Scientific Education and Training at MSK.
Uniting Experimentation and Computation
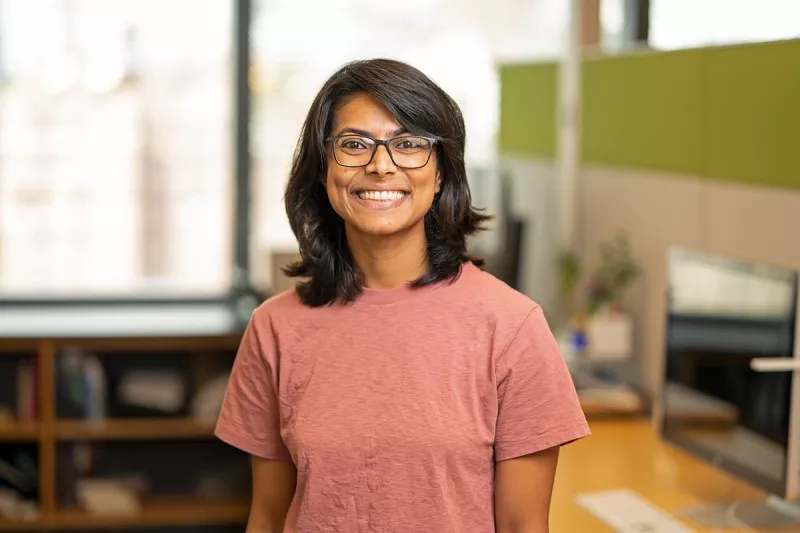
Sneha Mitra, PhD
Dr. Mitra’s project spans two labs: that of computational biologist Christina Leslie, PhD, and of Alexander Rudensky, PhD, who chairs the institute’s Immunology Program, where Dr. Mitra works closely with postdoctoral researcher Dan Feng, MD, PhD.
“There is a growing realization that complex biological systems, with their multitude of parameters, really cannot be understood without these advanced computational methods,” Dr. Rudensky says. He notes that the immune response in colorectal cancer involves a complex interplay of the tumor microenvironment, resident microbial communities, dietary impacts, inflammatory signals, and a host of diverse immune and other cell types.
Until fairly recently, he adds, theoretical or mathematical biology was considered a separate field from experimental biology, and the union between the two was focused on ensuring data models lined up neatly with laboratory observations. Computation-minded scientists were often treated as journeymen — like plumbers or electricians hired to do a job — and rarely as full scientific partners.
Today, however, as next-generation research technologies have become more pervasive, there has been a growing recognition of the importance of computational contributions to generating hypotheses and to the overall process of discovery.
“The new generation of cancer researchers will have a greater need to understand the principles of both experimentation and computation — the logic, the caveats, and being able to interpret results,” Dr. Rudensky says. “What we are witnessing is a movement of both fields closer together, and the need for researchers who are fluent in both worlds.”
‘I Would Never Have Had the Opportunity To Work in a Wet Lab’

Corey Weistuch, PhD
Marie-Josée Kravis Quantitative Biology Fellow Corey Weistuch, PhD, sees computational methods fueling 21st-century advances in biology.
“Biological experiments are costly in terms of time and labor and materials,” he says. “If we can tap into machine learning and use algorithms to quickly and inexpensively model the outcomes of experiments — what’s likely to work and likely not to work — we can accelerate the pace of discovery.”
Additionally, he says, computers can model some cellular and molecular processes that scientists aren’t able to measure directly with existing instruments.
Dr. Weistuch is using his fellowship to study liposarcoma, a soft tissue cancer known for complex DNA changes called copy number alterations, and for its devastating outcomes.
“Right now, liposarcoma has few good treatment options other than surgery,” says Dr. Weistuch, who holds a doctorate in applied mathematics and an undergraduate degree in math and biochemistry. “And unfortunately, it tends to recur and become fatal. It’s such a complex disease that to develop drugs against it, we first need to understand not only the mechanisms by which it grows and evolves but also to develop a computational framework to be able to characterize key differences within different types of cells across the same tumor.”
To do that, Dr. Weistuch developed an algorithm to model the accumulation of genomic amplifications and deletions within a liposarcoma tumor using information gathered through painstaking single-cell analysis of the diverse cells that make up these tumors.
“This helps us figure out which genomic changes came first, because there are often those early events that are really going to drive the progression of disease as well as drug resistance,” he says.
Ultimately, the goal is to identify vulnerabilities and novel approaches to target those early changes, paving the way for new targeted therapies.
Through the fellowship, Dr. Weistuch’s research is a collaboration, bridging the lab of Joseph Deasy, PhD, Chair of the Department of Medical Physics at MSK, and the lab of Samuel Singer, MD, Director of MSK’s Sarcoma Center.
“Dr. Weistuch has developed new algorithms to unravel the somewhat chaotic world of cancer evolution as revealed by measurements on individual sarcoma tumors,” Dr. Deasy says. “He has creatively borrowed ideas from statistical physics and optimal transport theory, as well as other areas, to develop these promising tools. Allen Tannenbaum, PhD, his other computational mentor, and I are excited to see what comes out of this wonderful collaboration with the Singer Lab.”
Moreover, the fellowship has allowed Dr. Weistuch to get out from behind the computer terminal and hands-on with human tumor samples and mouse models of liposarcoma while running experiments to help validate the accuracy of his predictive modeling on the computational side.
“Without this support, I would never have had the opportunity to work in a wet lab in the first place,” he says. “Not only did that give me new insights into experimental science and laboratory culture, but it also helped me learn more about the real-world properties behind the data to help develop a better algorithm.”