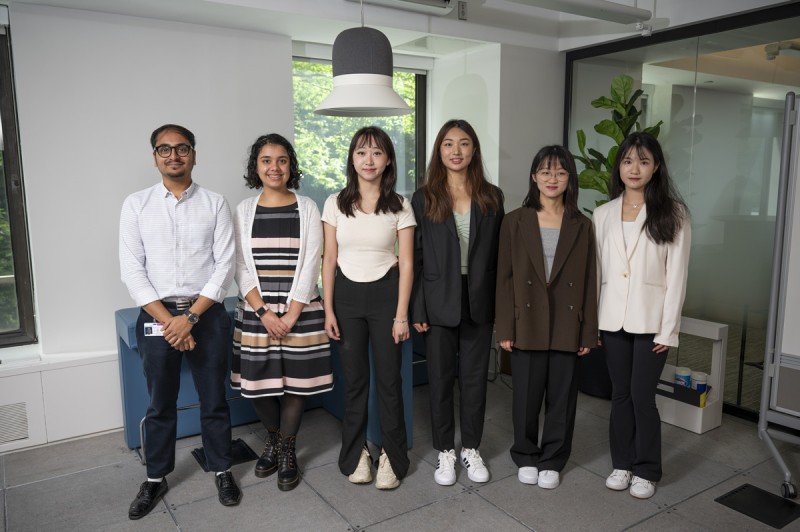
GEMS Class of 2023
Questions? Please send an email to GEMS@mskcc.org
Program Overview
Genomics Experience for Master’s Students (GEMS) is a 12-week program for master’s level quantitative scientists that aims to create an immersive experience for the student to engage in real-world team science projects and learn to apply and translate their quantitative skills into meaningful scientific contributions in cancer medicine with a focus on cancer genomics and precision oncology. This full-time, on campus research experience allows students to fully engage with mentors and a multidisciplinary research team on cutting-edge projects with the goal to propel them into genomics-oriented data science careers. Each fellow will have two mentors – one quantitative/computational mentor and one scientific mentor – to provide a highly interdisciplinary and immersive training environment and will prepare students for the interdisciplinary translational science workforce. Trainees must demonstrate a strong interest to learn cancer genomics and need not have experience in this area. The GEMS program is supported by the National Cancer Institute (R25-CA272282) and the MSK Society.
Program Goal
Through this immersive 12-week experience, fellows will gain:
- Perspective on modern frontiers in cancer genomics research
- Exposure to modern foundational concepts and methods taught through the seminar series
-
Knowledge of foundational concepts and methodology including:
- how to process and manipulate large multi-dimensional data
- methods for data normalization, dimension reduction
- detection and correction for batch effects
- software and tools for data visualization
- basic strategies for integrative analysis of multidimensional and multimodal data
- Education in the responsible conduct of research
- Training in effective scientific communication
- Experience in critical thinking, problem solving, and teamwork through the dual-mentorship model embedded in a multidisciplinary scientific team.
Important Information
Accepted fellows will be paid a modest stipend. Housing is not provided, but GEMS will provide a nominal payment for housing expenses. A portion of fellow travel to and from New York City is eligible for reimbursement.
Applications for Summer 2025 will open November 1st and close on January 21st.
The Summer 2025 program will run from May 19th to August 8th.
Eligibility Criteria
Eligible applicants must be:
- Currently matriculated in a master level program (biostatistics, statistics or related field)
- Trained in statistical theory, methods and programming and related fields
Application Requirements
- Resume
- Statement of interest (~500 words)
- Three letters of recommendation
Internship Location
The GEMS program is housed within Memorial Sloan Kettering’s Department of Epidemiology and Biostatistics, located in midtown Manhattan. The Department’s offices at 633 3rd Avenue are in easy walking distance of several New York City subway lines and the Metro North Railroad at Grand Central Terminal. Penn Station (Long Island Railroad, New Jersey Transit) and the Port Authority Bus Terminal are easily accessible via mass transit. MSK operates regular shuttle buses between midtown and MSK’s main campus on the Upper East Side, where GEMS fellows will have access to the MSK Library and to relevant lectures, seminars, and other educational activities.
Research Areas
Proposed projects will apply statistical and computational methods in areas including:
- Precision oncology approaches to optimizing cancer therapy and patient response
- Impact of mutational processes on immuno-suppression and evasion
- Single cell transcriptomics profiling for cancer immunotherapy
- Multi-omic characterization of cancer genomes and racial disparities
- Data science approaches to derive real-world evidence in oncology with integrated genomic and electronic health records data
2024 Projects
Dr. Teng Fei
Association between radiomic and genomic features in a CAR-T cohort
In this multi-omics investigation of a cohort of patients receiving CAR-T therapy, we would like to correlate radiomic and genomic features to obtain better understanding of the relationships between the two modalities. This exploratory analysis will attempt to explore various computational approaches to answer the research question, including pairwise feature correlations, network analysis, multi-omics integration, or others. The GEMS student will join a multi-disciplinary team of physician scientists, biostatisticians, and computational biologists to work in a collaborative environment.
Drs. Xiang Shu and Xinjun Wang
Breast cancer is a heterogeneity disease consisted of multiple subtypes with distinct biological characteristics and prognosis. The etiology and cell-of-origin for each subtype are not fully understood. The project aims to explore cell-of-origin for breast cancer subtypes including luminal A, B, Her2+, and triple negative cancer with application of cutting-edge tools for GWAS and single cell data analysis.
Dr. Yuan Chen
Cancer is a complex disease driven by genomic alterations, and tumor sequencing is becoming a mainstay of clinical care for cancer patients. The emergence of multi-institution sequencing data presents a unique and powerful resource for learning real-world evidence to enhance precision oncology. However, leveraging sequencing data from multiple institutions presents significant challenges. Variations in gene panels result in loss of information when the analysis is conducted on common gene sets. Additionally, differences in sequencing techniques and patient heterogeneity across institutions add complexity. High data dimensionality, sparse gene mutation patterns, and weak signals at the individual gene level further complicate matters. To address these challenges, we introduce a novel statistical model, referred to as Bridge, to effectively integrate and harmonize the multi-institution genomic data. When applied to the AACR GENIE BPC data, a global effort that generates a real-world, observational database linking genomic data with clinical information across institutions, the Bridge model consistently demonstrates superior prediction accuracy for survival across six distinct cancer types.
Dr. Xinjun Wang
Optimize the Computational Scalability of Model-based Clustering Methods for scRNA-seq Data
Single-cell RNA sequencing (scRNA-seq) is a rapidly developing technology that allows researchers to study gene expression at the single-cell resolution. In addition, the state-of-art droplet-based platform has several substantial advantages such as high throughput, improved accuracy, and allowing for multiplexing multiple samples in the same run to reduce cost and batch effect, which makes it a reliable tool and efficient method to advance our understanding of diseases and biology. Model-based clustering methods are popular in scRNA-seq. For example, DIMM-SC employs Dirichlet multinomial distribution to directly model the count data (i.e., UMI count) from scRNA-seq. We will compare the clustering accuracy as well as the computational speed between the new algorithm and the existing package, and also between EM algorithm and some gradient descent algorithms implemented in PyTorch.
Past Projects
- Identifying Fragile and Unstable Tregs using scRNA-seq
- Integrating and harmonizing multi-institutional sequencing data from GENIE BPC.
- Radiomics Associations with CAR-T Clinical Outcomes
- Building Multimodal Models for Predicting Survival and Recurrence After Liver Resection in Metastatic Colorectal Cancer Patients.
- Operationalizing a “Functional Potential Score” (FPS) To Proiritize GWAS Variants for Experimental Studies
- Evaluating Melanoma Cancer Therapies via scRNA-seq Profiling of Mice Samples
MSK is an equal opportunity and affirmative action employer committed to diversity and inclusion in all aspects of recruiting and employment. All qualified individuals are encouraged to apply and will receive consideration without regard to race, color, gender, gender identity or expression, sexual orientation, national origin, age, religion, creed, disability, veteran status or any other factor which cannot lawfully be used as a basis for an employment decision.